Advanced analytics: from data-driven to outcome-driven analytics
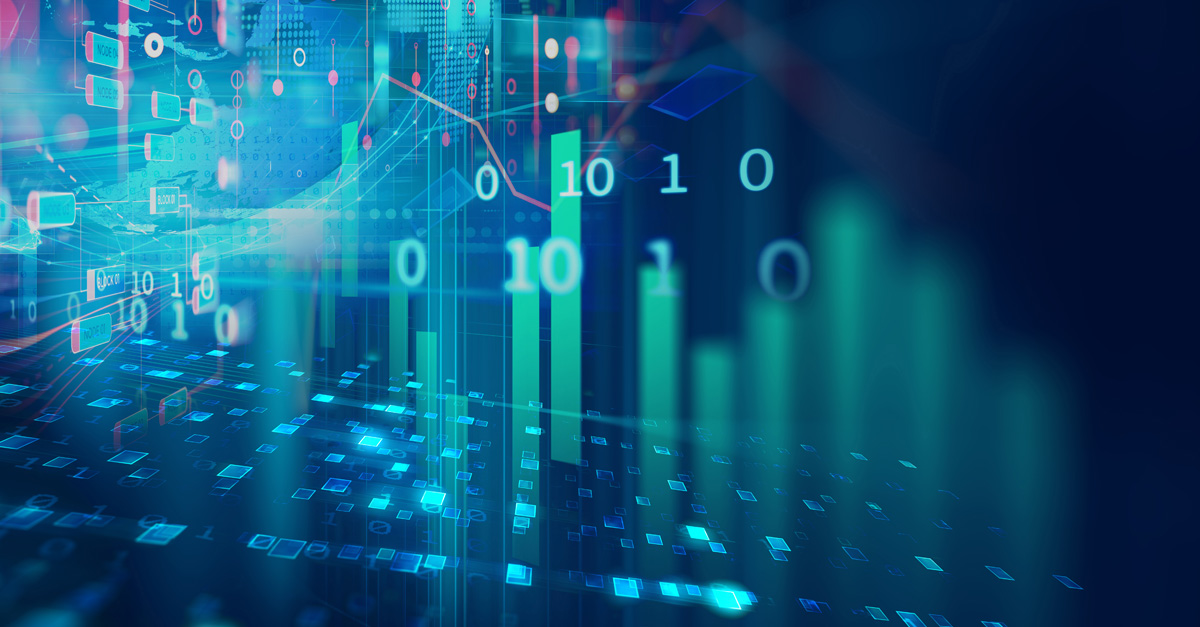
The obsession to know the future
Human beings have been and always will be obsessed with knowing the future with the wonder that if they know what will happen they can make better decisions and achieve better results.
In business, the obsession is the same. In the last 15 years of my working experience, I have seen, participated in, and delivered work on contributing to forecast modeling and analytics. What I learned is that as soon as the prediction is out, it is by definition not the truth nor the reality of the future, but rather an estimation of what will or might happen. Whatever the method, a prediction is just a prediction, that’s it. In best cases, it becomes a support for decision-making but it should not be the only criteria to make decisions.
Measuring robustness of predictions
In the search for the most accurate forecast, I have seen that another obsession is to find out how robust the calculation is, and how accurate the forecast model is. Those are valid and key concerns for managers.
However, in these discussions between business, IT and analytics specialists, I sometimes observed that the business problem we were trying to solve became a secondary issue. On the other hand, the prediction and its accuracy attracted all the attention. Of course, it is very important to discuss what makes a good predictive model and how to define accuracy and robustness. But this should not be the sole focus. After all, the question is: assuming the forecast model is robust, what will business people do with the predictions?
What matters are the outcomes, the desired outcomes!
With the time, a lot of intense discussions, and some self-reflection, I found out that what matters is the outcome of the business decision in which the forecasts and predictions are input contributions to the decision-making process. This is not the only contribution but an important one. So, I have reason to believe that the robustness of the predictive model is crucial, but not the only decisive element.
Unfortunately, I have only seen on rare occasions, mostly academic ones or experiments, that data based on the business decisions and their outcomes are collected, then exploited to further enrich not only the predictive models but also to measure the effectiveness of the decision.
And here is where I noticed a paradox: business people tend to focus on the robustness of the models and very little on the effectiveness of the decisions made based on the forecasts.
Focus on the end-to-end
So, when implementing an advanced analytics solution (Proof of Concept / PoC, Proof of Value / PoV, reports, you named it), the recommendation would be to focus on the end-to-end process and a limited scope of the business rather than solely on the forecast model piece.
Maybe, for the end-to-end approach, additional data will be required to feed the model. If so, the scope can be limited, so that the efforts can be efficiently and effectively invested. The key idea is to quickly decide whether or not the approach is the correct one before scaling the models and technical solutions. The reverse would be to use the “big data” already available for predictions to make decisions, and not measure the results, but focus on the accuracy of the predictive model.
Learn, learn, and learn
Let us take a fictive example based on the “holy grail” topic in the supply chain, OTD (on-time delivery). Everyone in the supply chain wants to know the OTD rates. Here no issue, information is based on past performance. And everyone wants to know what the future OTD of the suppliers will be, what we can also call ETA (estimated time of arrival). In simple terms: when will my parts arrive?
In this context, knowing the prediction of your orders’ ETA can allow you to identify the deliveries at risk and decide what to do: escalating towards your suppliers to put additional priority on your order may seem to be the natural reaction. After all, you are an important customer and by letting know your suppliers that the order at risk is important, your suppliers could do their best and shifts priorities.
So, you got it! Securing the arrival of the ordered parts on time is the desired outcome. In this example, the predicted ETA is important, but also the decision to act plays a role. As soon as the action was taken, this information should also feed your predictive model and adjust the prediction. The question is, are you gathering this data? And what is more important, did you manage to get the parts delivered on time? If the answer is yes, your decision might have been the right one (at least this time). Will it work the next time? Do you know how your business decision impacted your suppliers’ operations or the rest of your orders etc…
If you can get all this data in your model, you’d be able to measure the results at an aggregated level and see if the model and the decision you made will deliver the desired outcome. The result can be interesting (see Graph 1).
After the implementation of the ETA forecast model, a possible outcome could look as follows:
- The percentage of suppliers with OTD performance above 60 % has decreased while the percentage of suppliers with an OTD below 60 % has increased.
In the previous example, where we focused on an individual order, we have had the impression that the outcome was the right one. But on an aggregated level, after prioritizing based on the ETA forecast, it seems that prioritizing the orders led to an outcome where the overall performance of your suppliers did not improve.
What happened?
- The prioritization might have worked out for some deliveries identified as at risk, but it might have caused disruptions for other orders.
So, on an individual order basis, there is a risk of getting biased and assuming that the prediction model was right: “I took action and the supplier was good at prioritizing my order, so I got my order on time. Great job.”
And for the orders that got lower priority, we would be tempted to say that the prediction model was right: we knew this order was about to arrive late, and so it did.
To avoid such a “quick and dirty” conclusion, we should delve deeper. In Graph 2, you see the results of what happened for the orders concerning standard parts. The result is quite similar at the aggregated level. In simple words, prioritization did not raise the overall OTD performance. Moreover, it worsened the situation for orders with an above 60% OTD.
We observe in Graph 3 that actually the OTD performance for customized parts improved. The percentage of these orders with OTD performance above 60% has increased:
So, we’d be tempted to say it worked out. And actually, it did.
In this example, we can deduct that for orders concerning customized parts, requesting the suppliers to prioritize the orders can have a positive effect on OTD. This might be due to the fact, that production lines for customized parts are more flexible than production lines for standard parts. So prioritizing orders for standard parts could be more challenging for the suppliers. Or maybe there is another reason for this and further analysis might still be required.
As matter of fact, the key learning is that not only the accuracy of our predictive model should be assessed and that the business decision might play a bigger role in the outcome than initially assumed. Our lesson learned here would be: to track the outcomes and assess the business decisions made before and after the implementation of predictive tools.
Conclusion: Focus on the desired outcome and track not only the prediction’s accuracy but also your business decisions!
- There is nothing wrong with forecasting and predictive models, just ensure it’s not your only focus.
- Focus on the desired outcome. Make your data and the predictive model work for you. Have an end-to-end approach.
- If there is not enough data in your systems, start small and enrich your model to keep track of the results of business decisions. Later on, this can be used to extend your model and start considering a prescriptive model.
- Measure all the results and find out in which cases the model and your decisions work and when not. Do not get biased by focusing only on the positive outcome.
- Advanced analytics might be a good recipe to help support your business issues, as there is currently still no one size fits all solution.
- Analytics, big data techniques and technology might have developed exponentially, however there is still a way to go to exploit them maturely — so keep learning from your data and your business.
- Next time you are considering requesting your suppliers to prioritize your orders at risk, think twice!
If you are interested in knowing more, about how SupplyOn supports its customers with advanced analytics, contact me, my sales colleagues, or our analytics experts. We would be happy to help you!