Predictive delivery date: AI predicts production completion and delivery time
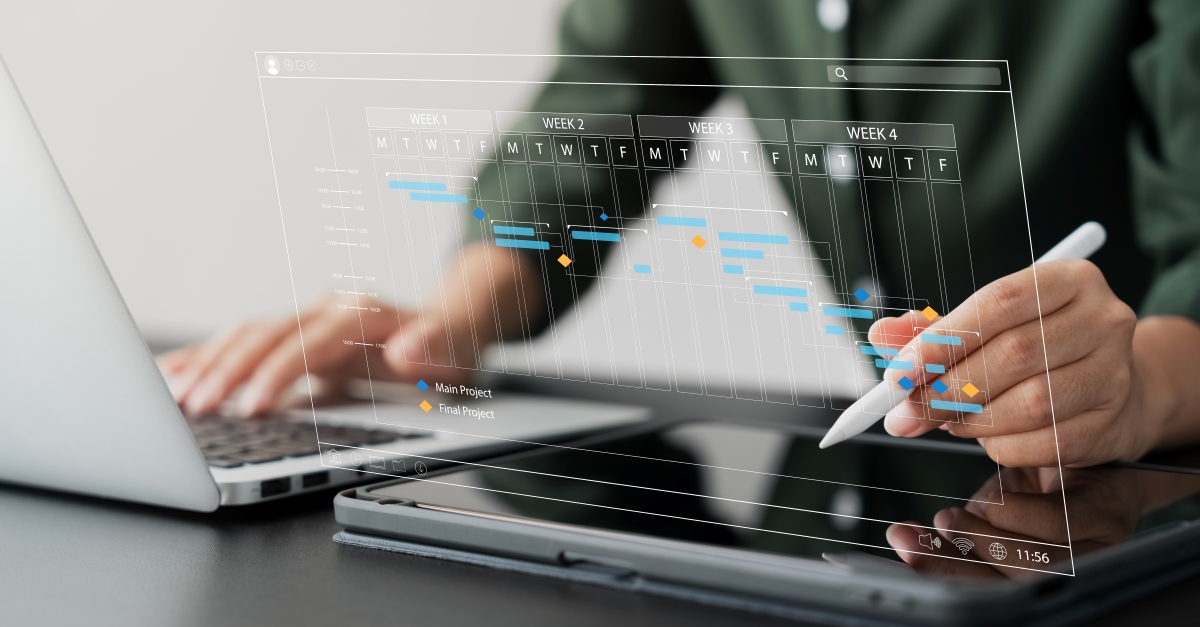
Production processes can be severely disrupted when it is only discovered on the scheduled delivery date that the ordered production material will not arrive on time. Since this material often travels through long and complex supply chains consisting of many planning, ordering, production, and delivery processes, bottlenecks are not uncommon in practice. The problem is exacerbated by the fact that modern manufacturing plants often have very low inventory levels, meaning that materials are delivered just prior to production.
It is therefore particularly important that production and material planners are informed as early as possible of any potential delivery problems. Only then can timely action be taken, such as ordering a replacement or rescheduling production.
Shortly before delivery, the goods are in transit. During this time, the carrier closely tracks and monitors the status of the shipment. However, modern transportation times can be very short, and notification of delivery delays often comes too late to take countermeasures.

Insight into supplier production
The solution is to identify discrepancies in production planning and production at the supplier long before transportation. SupplyOn automatically collects a wide range of standardized production data from the supplier. This makes it possible to track which production quantities are in the planning phase and which are actually in production. The transmitted data also makes it possible to monitor production progress and determine which specific production step the order is in. This provides visibility down to the sub-assemblies and raw materials used.
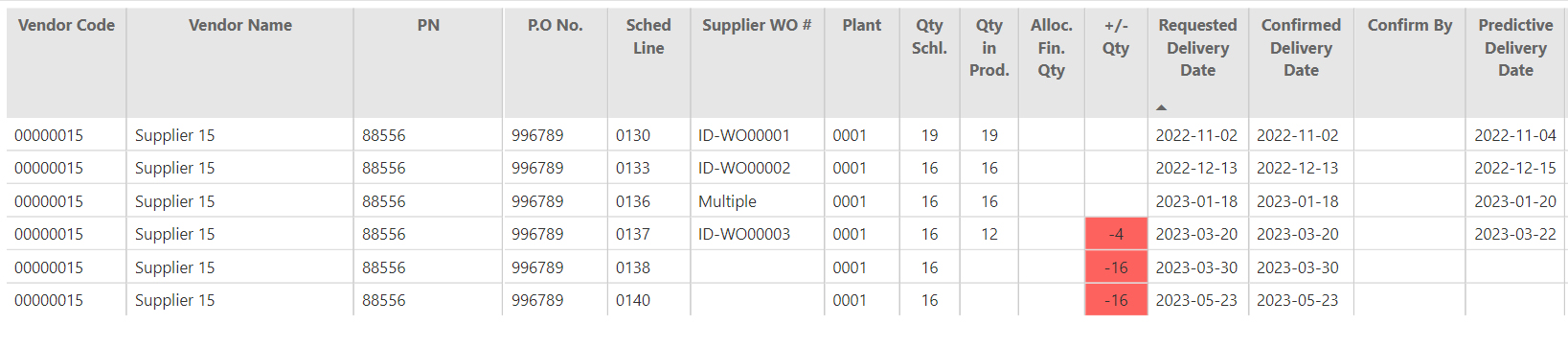
The power of our production-to-supply solution is that quantities in production can be mapped directly to buyer requirements. This provides immediate visibility into which requirements are already scheduled with the supplier or are already in production. By constantly monitoring the progress of work, the AI-powered system automatically learns normal production lead times and also recognizes when current production is slower than usual.
Using AI to spot variances before they become a problem
A complex, continuously learning predictive model based on historical monitoring data calculates an estimated completion date, taking into account recently observed production times and current work progress. This makes it possible to estimate, either during production or before production begins, whether the manufactured goods will be ready for shipment and whether the desired delivery date can be met.
The highly AI-driven system generates early warnings for various situations:
- The planned production quantity is too low
- The planned production start is too late
- The quantity in production is insufficient to meet the upcoming demand
- The production start is too late or will be delayed to meet the delivery date
Based on the forecast of the current production time, it is also possible to determine when the production for the upcoming demand has to start in order to avoid delivery delays. The system also records the difference between the quantity produced and the quantity delivered. This can be used to determine the percentage of scrap and to calculate a recommended production quantity in addition to the recommended start of production.
In addition to clear graphical and tabular displays, the predicted delivery date, recommended production start and recommended production quantity can be sent directly to the customer’s ERP system. This means that the AI system’s calculations are available to the customer at the line item level.
Optimized planning at both ends of the supply chain
By combining demand and production data and performing intelligent calculations, the system provides valuable insights to both the purchasing and supply sides, enabling both to improve their planning processes. This increases the likelihood that production will start on time and in sufficient quantity.
Production delays are automatically detected and alerts are generated, providing insight into exactly where production is stuck. This allows for timely and targeted adjustments
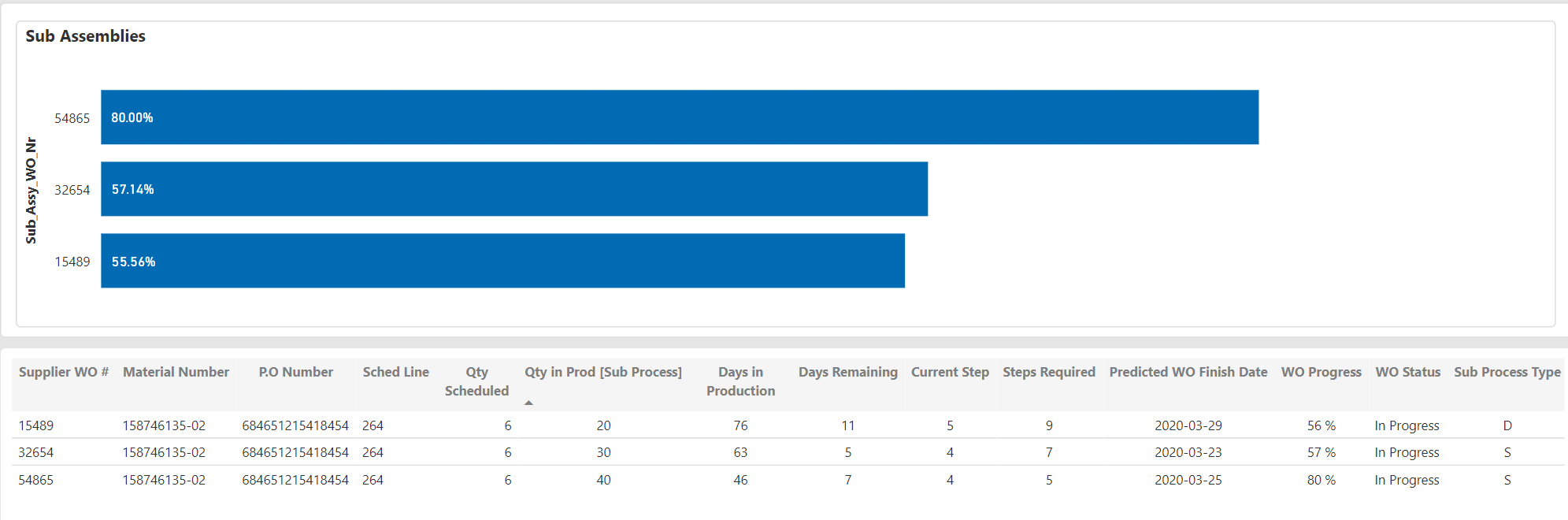