Smart Logistics Data: the intelligent supply chain
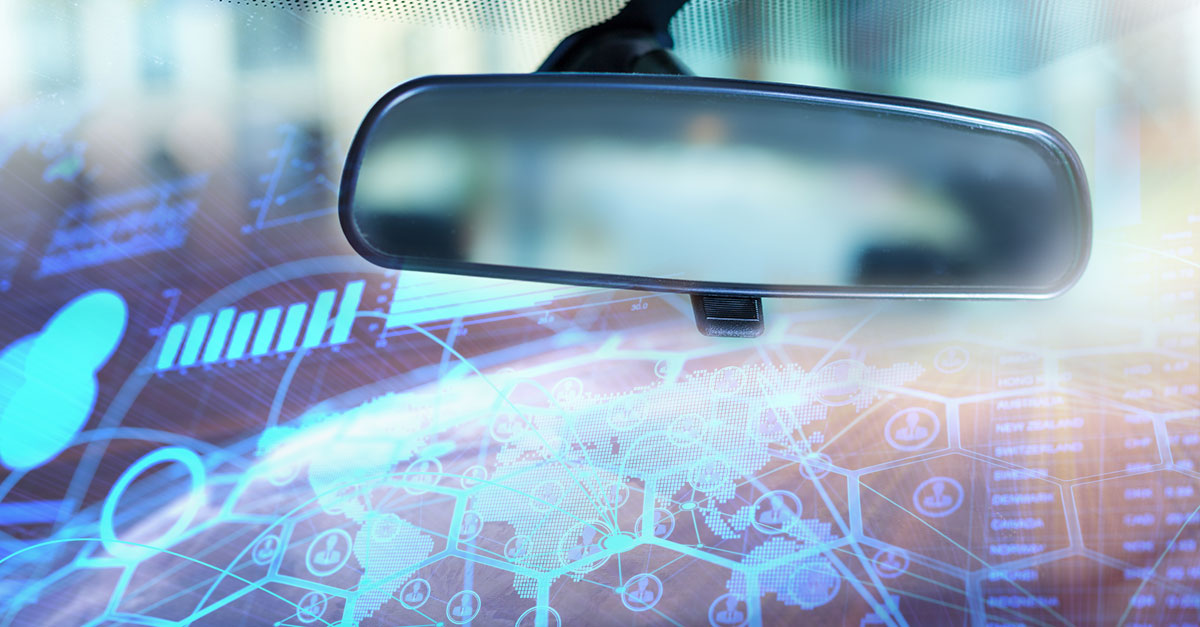
A supply chain has to be agile, robust and resilient. Capable of anticipating potential risks and responding in advance, detecting problems early on and flexibly circumventing them.
All this requires the intelligent use of data. But how can we really make data “smart”?
Obstacle 1: Data problems wherever you look
If we take a look at the typical supply chain process, the first thing we see is not “smart” data but a whole lot of data problems. Usually the data are hidden away in countless silos – the ERP system, various SaaS solutions and possibly other systems we can’t even access. In short: the data are distributed between different areas subject to differing control.
And the data aren’t just spread out over different technical systems, they’re heavily fragmented, too. Some data are to be in the area of supplier management, some are in supply chain management and some in transport management.
In addition, there is a lack of real-time data. Data exchange between collaboration partners is usually asynchronous and takes place at substantial time intervals.
Finally there is a lack of data harmonization. For example, we generally have to struggle with differing material numbers for one and the same item as it moves along the supply chain. The same applies to component numbers, for instance.
Obstacle 2: Driving forwards while looking backwards – with a blind rear mirror
This makes it virtually impossible to get a comprehensive view of the entire range of data involved in a supply chain process – let alone engage in proactive management when problems arise. And a whole lot of problems can potentially arise in the course of the supply chain process. Starting with the order itself: some suppliers don’t even confirm it, while others do so with completely different order quantities or delivery dates. A lot of companies are not familiar with the capacity limits of their suppliers and request much higher quantities than the latter are capable of producing.
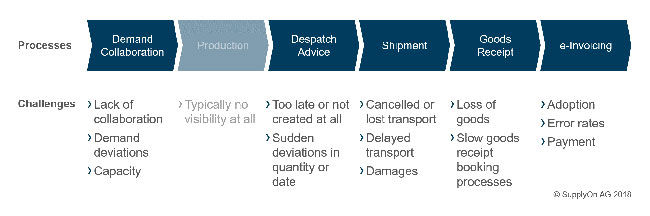
During the next stage of the process – production itself – the company placing the order is completely blind. The problem is that this process step can take anything from weeks to months in the manufacturing industry.
Not until the shipping notification has been issued does the ordering company get an insight into the process again – if the supplier does actually issue one. In practice, the shipping notification frequently differs from the original order – an unpleasant surprise for the customer. And one which gives him barely any time to respond.
And so the potential problems continue: from delayed, lost or damaged items and uncertainties when goods are received through to errors in electronic invoices.
Even the best reporting system or use of classic business intelligence are not much help here: this is because they don’t look into the future but merely document problems in the past. It’s like looking into a rear-view mirror. And even the rear-view mirror frequently only shows us individual pieces of the puzzle: some pieces are missing completely while others are lacking the connecting parts, so it’s not clear at all how they fit together.
If, however, it’s only possible to analyze the individual process steps separately, it is almost impossible to investigate and eliminate the causes. This is all the more true if some process steps are missing completely and data only comes in intermittently.
How can you generate a coherent overall picture if you only have some of the pieces? A picture that is updated and extended in real time? A picture that looks forward rather than backwards?
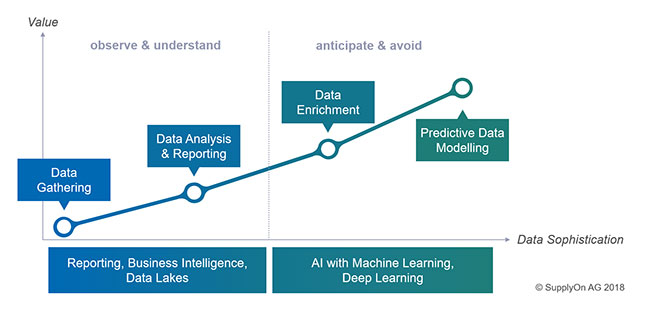
Solution – Part 1: Enhance the data
The only way to achieve this is by means of data enhancement. We have to analyze data in their context – and to do this we must tap into data sources that can give us additional information about the data we already have.
In concrete terms, this involves information from a wide range of different sources flowing into a so-called data lake. Firstly this will be the data from the customer side, usually stored in ERP systems – forecasts, order details, call-offs and goods-receipt information.
Then the data lake is fed by a range of information from the supplier side such as master data, logistics data (order confirmation, forwarder pickup advice [FPA], shipping notification etc.) and requests for quotation.
In addition there is information from the transport management system. This will typically include status reports such as On-Time Delivery (ODT), Estimated Time of Arrival (ETA) and the shipment number. Nowadays we can also integrate even more detailed transport information such as precise location data, information about the condition of the transported goods and even the actual freight costs.
Above and beyond this more or less classic information, we can also use APIs to draw on a wealth of additional data. Key elements here would be information about the supplier’s production process. The latter’s capacity, stocks, start of production and volume output give us important data that eliminates what have up to now been blind spots, enabling us to detect any potential planning errors on the supplier side early on.
But there is still a wealth of other relevant information we can use. This includes IoT sensor data, risk information regarding strikes and political situations and even weather data that might impact on our transport on certain routes. And finally, social media gives us a valuable source of data from which to gain useful insights into events relevant to the supply chain.
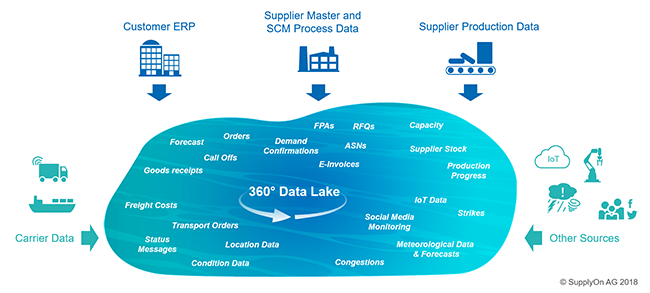
Solution – Part 2: Breathe intelligent life into the data
All these data give us crucial parts of the puzzle which we can piece together to create a comprehensive picture and look into the future. But for this purpose we need intelligent models that are based on the data – in other words: predictive analytics.
Based on machine learning, deep learning and other methods, artificial intelligence then enables us to achieve a whole new dimension of data quality so as to anticipate future problems at an early stage. Or if you like: it gives us smart data in the true sense of the word.